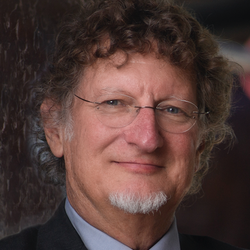
Optimally Pooled COVID-19 Testing
A Talk by Prof. Dor Ben-Amotz (Purdue University, Department of Chemistry West Lafayette, IN, USA)
About this Talk
Dor Ben-Amotz, Purdue University, Department of Chemistry, West Lafayette, IN, USA
It has long been known that pooling samples may be used to minimize the total number of tests required in order to identify each infected individual in a population. Here such predictions are extended to yield practically useful guidance regarding of the range of infection rates over which a given fixed pool size remains nearly optimal, as well as the significant additional testing efficiency that may be obtainable using a second round of pooling for populations with infection rates below 10%. Binomial statistics are used to predict optimal first and second round pool sizes that optimize testing efficiency by minimizing the total number of tests needed in order to identify each positive individual in a population with a given average infection rate, as well as the range of infection rates over which a particular pool size remains near-optimal. The results yield the optimal first and second round pool sizes required to most efficiently test populations with infection rates ranging from 0.1% to 30%. The predictions are validated using recently reported COVID-19 (SARS-CoV-2) pooled testing and detection sensitivity measurements performed using both near-optimal and non-optimal pool sizes, and are further used to quantify the additional improvement in testing efficiency that could have been obtained using optimal pooling. For example, for a population 100,000 with an infection rate of 1% it is predicted that only about 15,000 standard RT-qPCR tests for SARS-CoV-2 should be required to identify every infected individual, using two rounds of pooled testing with optimal pool sizes of 11 and 4, respectively. If only the first round of optimal pooling had been performed then 20,000 tests would be required, while non-optimal pooling with a pool size of 5 would have required about 25,000 tests. Although a large pool size near 30 is predicted to be most advantageous for testing populations with very low infection rates near 0.1%, such large pool sizes are predicted to become highly non-optimal with increasing infection rate, while pool sizes smaller than 10 remain near-optimal over a broader range of infection rates.