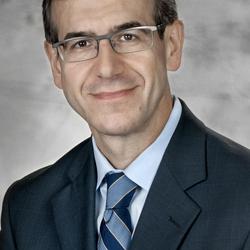
Spatiotemporal analysis of micro-epidemics of influenza and COVID-19
A Talk by Prof. Eleftherios Mylonakis (Alpert Medical School of Brown University, Rhode Island Hospital & The Miriam Hospital, USA)
About this Talk
Authors: Evangelia K. Mylona, M.Eng.1, Fadi Shehadeh, M.Eng.1, Markos Kalligeros, M.D.1, Gregorio Benitez1, Philip A. Chan, M.D. 1,2, and Eleftherios Mylonakis, M.D., Ph.D.1
Affiliations: 1Infectious Diseases Division, Warren Alpert Medical School of Brown University, Providence, RI, USA, 2Rhode Island Department of Health Division of Preparedness, Response, Infectious Disease and EMS (PRIDEMS)
Abstract: Infectious disease outbreaks and epidemics are facilitated by social networks and micro-communities. Real-time interventions can break the transmission cycle public health surveillance is essential for monitoring and evaluating disease spread.
Seasonal influenza is a significant infection and represents a typical example of a seasonal epidemic, which occurs on a yearly basis and poses a major health burden. Similar to influenza, COVID-19 often presents as an acute respiratory syndrome and the role of hospitals and healthcare networks, in monitoring micro-epidemics, super-spread events and hot spots, has become paramount. Understanding the clustering of cases in a detailed level is crucial for epidemic surveillance, and geo-referenced case data are needed for the implementation of early warning systems
We are utilizing laboratory confirmed influenza cases from a large healthcare network in Rhode Island (RI), US, in an effort to identify spatial and temporal patterns of epidemic spread at the community level. Then, we use the data from influenza epidemics to identify influenza spread patterns that could help focus resources and enact targeted micro-interventions in the places most needed. Moreover, we implement this methodology to identify and study spatiotemporal patterns of COVID-19.